Data Wrangling for Generative AI: Preparing Your Data for Success
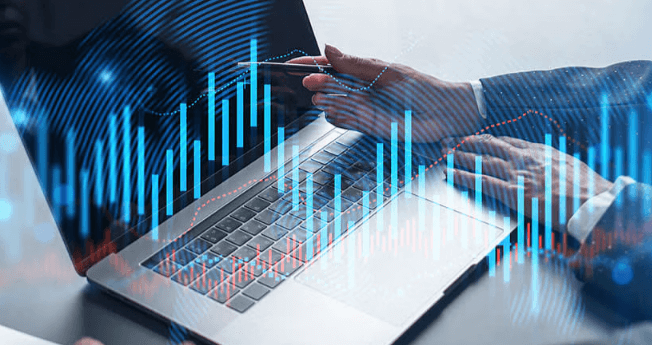
Data wrangling is an essential step in the development of generative AI models. It involves cleaning, structuring, and converting raw data into a format perfect for analysis and model training. Given the complexity and volume of data required for generative AI, effective data wrangling is critical for the success of any AI project. For those interested in mastering these skills, an AI course in Bangalore offers comprehensive training in data wrangling techniques, ensuring students can prepare data effectively for generative AI applications. This article explores the critical aspects of data wrangling for generative AI.
Understanding the Importance of Data Wrangling
Data wrangling is the foundation upon which successful generative AI models are built. Raw data is often critical, incomplete, and inconsistent. Before feeding this data into a generative model, it must be cleaned and preprocessed to ensure accuracy and reliability. Enrolling in an AI course in Bangalore provides hands-on experience with real-world datasets, teaching students the importance of data wrangling and its impact on model performance. By learning how to clean and prepare data, students can significantly enhance the effectiveness of their AI models.
Data Cleaning: The First Step
The initial step in data wrangling is cleaning the data. It involves handling missing values, correcting errors, and removing duplicates. In generative AI, where models require vast amounts of high-quality data, even minor errors can lead to significant performance issues. An AI course in Bangalore often includes modules on data cleaning techniques, ensuring that students understand how to identify and rectify common data issues. This foundational skill is crucial for aspiring AI professionals, as clean data is the bedrock of successful AI models.
Data Transformation and Normalisation
Once the data has been cleaned, the next step is to transform and normalise it. It includes converting data into a consistent format, scaling numerical values, and encoding categorical variables. These transformations ensure that the data is in a form suitable for model training. A generative AI course typically covers various data transformation techniques, such as normalisation and standardisation, providing students with the tools to preprocess data effectively. Understanding these techniques ensures that generative AI models can learn patterns from the data efficiently.
Data Integration and Enrichment
Data integration involves amalgamating data from different sources to create a comprehensive dataset. This step is crucial for generative AI, which thrives on diverse and rich datasets. Data enrichment, on the other hand, involves enhancing the dataset with additional information, such as external data sources or derived features. A generative AI course often includes practical data integration and enrichment exercises, teaching students how to merge datasets and add valuable context to their data. These skills are crucial for building strong and versatile generative AI models.
Handling Large Datasets
Generative AI models require massive data to operate effectively. Handling such large datasets can be challenging, particularly regarding storage and processing power. Techniques such as distributed computing and cloud storage can help manage these challenges. A generative AI course often provides insights into handling large datasets, including using cloud-based tools and distributed computing frameworks. By learning how to manage and process large datasets, students can ensure that their generative AI models can access the data they need without being constrained by hardware limitations.
Ensuring Data Quality and Consistency
Data quality and consistency are paramount for the success of generative AI models. Inconsistent or poor-quality data can cause biassed or inaccurate models. Ensuring data quality involves continuous monitoring and validation of the data throughout the data-wrangling process. A generative AI course emphasises the importance of data quality assurance, teaching students various techniques for validating and maintaining high data standards. Students can build more reliable and trustworthy generative AI models by prioritising data quality.
Read also Real Estate Experts Share Tips for First-Time Homebuyers
Leveraging Automation Tools
The data-wrangling process can be time-consuming and labour-intensive. Leveraging automation tools can streamline this process, allowing data scientists to focus on more critical tasks. Tools like Python’s Pandas, NumPy, and specialised AI libraries can automate many aspects of data wrangling. An AI course in Bangalore often includes training on these tools, showing students how to use them effectively to automate data cleaning, transformation, and integration tasks. Students can significantly enhance their productivity and efficiency in data wrangling by mastering these tools.
Addressing Ethical Considerations
Data wrangling for generative AI also involves addressing ethical considerations like privacy and bias. Ensuring the data is ethically sourced and processed is crucial for building responsible AI systems. An AI course in Bangalore often includes modules on ethical AI practices, teaching students how to handle sensitive data and mitigate bias. Understanding these ethical considerations is essential for developing effective, fair, and responsible generative AI models.
Conclusion
Data wrangling is a critical step in the development of generative AI models. It involves cleaning, transforming, integrating, and enriching data to ensure it is perfect for model training. For those looking to develop expertise in this area, an AI course in Bangalore provides comprehensive training in data wrangling techniques, offering hands-on experience with real-world datasets and teaching best practices for data preparation. By mastering the art of data wrangling, AI professionals can significantly enhance the performance and reliability of their generative AI models, paving the way for innovative and impactful AI applications.
For More details visit us:
Name: ExcelR – Data Science, Generative AI, Artificial Intelligence Course in Bangalore
Address: Unit No. T-2 4th Floor, Raja Ikon Sy, No.89/1 Munnekolala, Village, Marathahalli – Sarjapur Outer Ring Rd, above Yes Bank, Marathahalli, Bengaluru, Karnataka 560037
Phone: 087929 28623
Email: enquiry@excelr.com